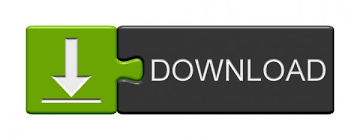
These residuals serve as indicators of the interaction term in the structural model. Orthogonalization: This approach uses residuals that are calculated by regressing all possible pairwise product terms of the indicators of the latent predictor and the latent moderator variable (i.e., product indicators) on all indicators of the latent predictor and the latent moderator variable. These latent variable scores are saved and used to calculate the product indicator for the second stage analysis that involves the interaction term in addition to the predictor and moderator variable. Two-stage (default): This approach uses the latent variable scores of the latent predictor and latent moderator variable from the main effects model (without the interaction term). These product terms serve as indicators ("product indicators") of the interaction term in the structural model. Product Indicator: This approach uses all possible pair combinations of the indicators of the latent predictor and the latent moderator variable. Selects the method of interaction term construct in PLS path modeling. Moderator Variableįield to define the moderator variable for which a moderating effect will be estimated. Predictor Variableįield to define the predictor variable for which a moderating effect will be estimated. The selected dependent variable for which a moderating effect will be estimated. Moderation Settings in SmartPLS Basic Settings Dependent Variable (2022) describe PLS-SEM moderator analysis in more detail. The following figure shows the conceptual model of such a moderating relationship, which only focuses on the satisfaction-loyalty link in the corporate reputation model. In such a setting, we would examine if and how the respondents’ switching barriers influences the relationship. These considerations also apply for the relationship between CUSA and CUSL in the corporate reputation model example. In the prior example, we hypothesized that only the satisfaction-loyalty link is significantly influenced by income. The testing of the moderating relationship depends on whether the researcher hypothesizes whether one specific model relationship or whether all model relationships depend on the scores of the moderator. Moderating relationships are hypothesized a priori by the researcher and specifically tested. As such, moderation can (and should) be seen as a means to account for heterogeneity in the data. Thus, this relationship is not the same for all customers, but instead differs depending on their switching barriers. In other words, switching barriers serve as a moderator variable that accounts for heterogeneity in the satisfaction-loyalty link. More precisely, switching barriers have a pronounced negative effect on the satisfaction-loyalty relationship: the higher the switching barriers, the weaker the relationship between satisfaction and loyalty. For example, prior research has shown that the relationship between customer satisfaction and customer loyalty differs as a function of the customers’ switching barriers. The moderator variable (or construct) changes the strength or even the direction of a relationship between two constructs in the model. Moderation describes a situation in which the relationship between two constructs is not constant but depends on the values of a third variable, referred to as a moderator variable. As an example, the relationship between two constructs is not the same for all customers, but differs depending on their income. In other words, the nature of the relationship differs depending on the values of the third variable. When moderation is present, the strength or even the direction of a relationship between two constructs depends on a third variable.
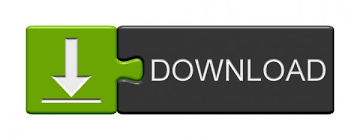